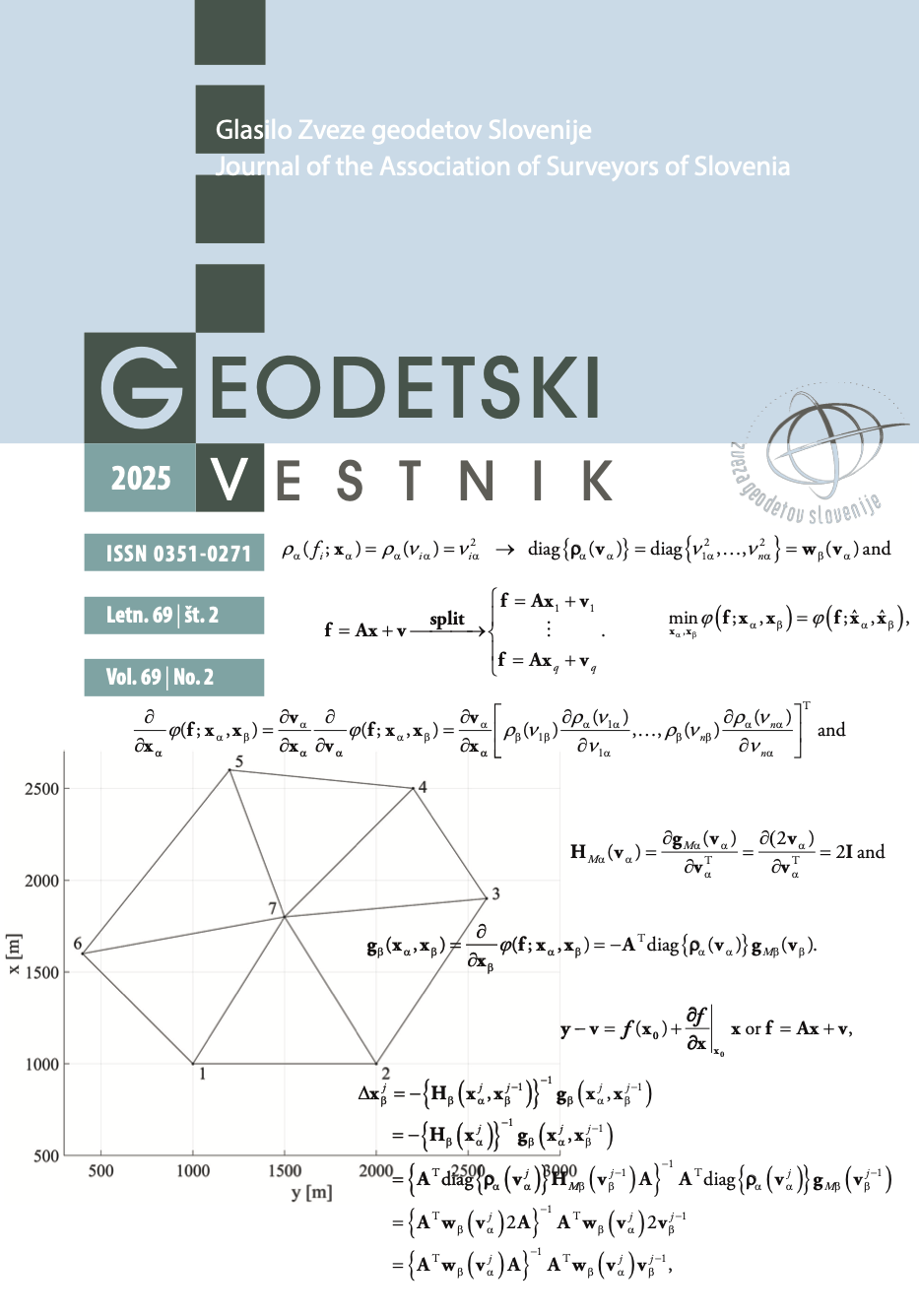
IF JCR (2023): 0.4
IF SNIP (2023): 0.487
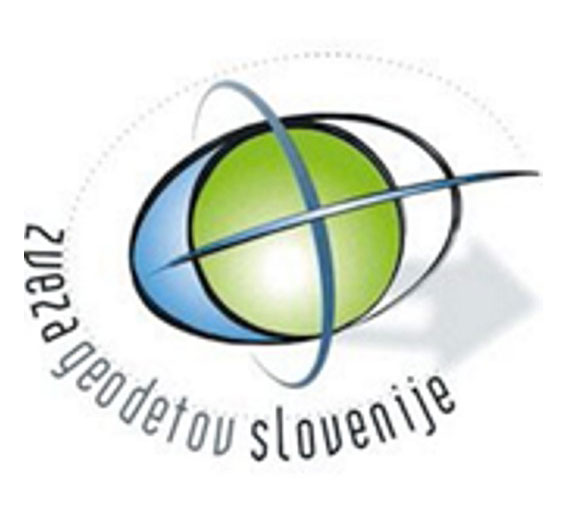
Association of Surveyors of Slovenia
Zemljemerska ulica 12, SI-1000 Ljubljana
E-mail: info@geodetski-vestnik.com
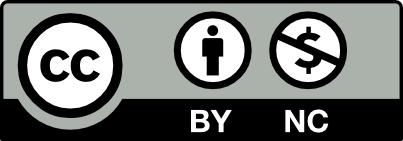
Geodetski vestnik
Geodetski vestnik is the publication of the Association of Surveyors of Slovenia (Zveza geodetov Slovenije) and it is an open access journal, issued quarterly in print and online versions. The journal is designed to serve as a reference source and an archive of advancements in the disciplines that make use of the geodetic, geospatial and spatial planning fields.
Current issue:
2025 (69), 2
Editorial
Peer reviewed articles
Žan Pleterski, Tomaž Ambrožič, Admir Mulahusić, Nedim Tuno, Jusuf Topoljak, Amir Hajdar, Adis Hamzić, Muamer Đidelija, Nedim Kulo, Gašper Rak, Aleš Marjetič, Klemen Kregar
115-147Squared Msplit Estimation in Deformation Analysis – 2D Geodetic Network Case Study
DOI: 10.15292/geodetski-vestnik.2025.02.115-147Klemen Kozmus Trajkovski, Tilen Urbančič, Bojan Stopar, Dejan Grigillo
148-179Photogrammetric traverse for indoor positioning
DOI: 10.15292/geodetski-vestnik.2025.02.148-179Mihaela Triglav Čekada, Edo Kozorog, Božidar Premrl
180-204The Jama (Luegg) estate boundary marks in the forests of Hrušica, Slovenia
DOI: 10.15292/geodetski-vestnik.2025.02.180-204Le Hung Trinh, Kim Thu Le, Van Phu Le
205-219Spremljanje sprememb mestnih zelenih površin v Vietnamu z uporabo podatkov Sentinel 2
DOI: 10.15292/geodetski-vestnik.2025.02.205-219Professional discussions
Matjaž Mori
221-224Local Solutions with Open-Source QGIS, Presentation of AGIS, SiKataster and ZUMbox Plugins
Tomaž Podobnikar
225-233High quality spatial data: reality or illusion?
Tomaž Petek, Ksenija Mihajlović
234-240Assignment of new generalized values of real estates
News from the field
241-246
- Ksenija Mihajlović idr.: Novice Geodetske uprave Republike Slovenije
247-258
- Anka Lisec: Novice s FGG
- Maja Filač: Strokovna ekskurzija študentov MA GIG in PN
- Neja Flogie: Terenski del izvedbe predmeta Projektno delo v kartografiji
- Kristina Korošec in Urša Švigelj: Opredelitev prostorskih potencialov Goriške razvojne regije za razvoj odgovornega turizma prihodnosti
- Geodetski inštitut Slovenije: Evropsko tekmovanje o obdelavi velikih Podatkov (European Big Data Hackathon)
News from societies
259-264
- 53. Geodetski dan: Geodezija in energetika: skupaj v prostoru, najava in program
265-282
- Milan Brajnik: Kamnolom skrivnosti
- Milan Brajnik: Geodetski izlet spregledana Srbija
- Tomaž Cink in Saška Kramar: Jubilejna Portugalska
- Jožica Marinko: Izlet Društva geodetov Gorenjske na Notranjsko
- Samo Vetrih: Ekskurzija Društva študentov geodezije Slovenije v Budimpešto
Miscellaneous
Ads
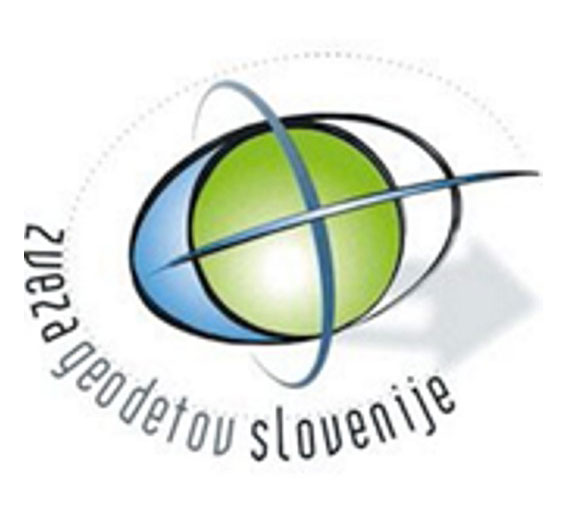
Association of Surveyors of Slovenia
Zemljemerska ulica 12, SI-1000 Ljubljana
E-mail: info@geodetski-vestnik.com
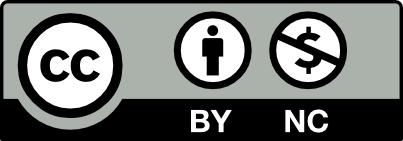